Academic Schedule Review for Energy Savings Potential
July 3, 2023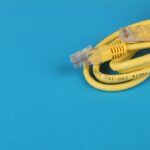
Guidelines for Choosing IOT Networks in the US, EU, and UK
July 12, 2024By Sam Kome, SRT Labs Technology Partnerships Manager
originally posted on LinkedIn, July 5 2024
Artificial Intelligence (AI) and Machine Learning (ML) are as prevalent in smart city conversations as anywhere. In April 2024, I met with engineering students visiting North Carolina State University from Saudi Arabia to engage our future leaders because (1) they will be building the systems for smart cities, and (2) they haven’t formed their visions yet of what a smart city would be and it is important that until it is nailed down, we realize the complexity of building something not yet fully defined. Collectively, we defined a smart city as one that would use data, networks, and other technology to solve problems for its residents. (As good a definition as can be imagined, actually!)
What is Common Across a Smart City?
“City” implies a more dense urban environment. While the geography may present some unique challenges, the infrastructure is ubiquitous: commercial and residential buildings are present, and the systems that reside in buildings: HVAC, lighting, water, waste, electrical, custodial, and access control.
But all of these building systems can also be assessed and optimized through the lens of occupancy, or how people are present in and using the buildings. At SRT Labs, we have scheduled HVAC temperature set points and a fresh air circulation program according to occupancy sensor data, air quality sensor data, and a room schedule system, to save energy and air filter changes. This relatively simple combination of data—with some rules—can bring significant improvements. As we layer in rules, the question becomes, how does AI or machine learning improve on rules-based routines?
AI/ML to Improve Rule-Based Routines
Definitions of “AI” are as plentiful as those for “smart city”. They run the gamut from what would usually be called machine learning to neural networks, to generative AI, to the so-far unattainable general artificial intelligence. Machine learning (ML) has been looking for work in smart buildings and cities for a long time. Typical examples focus on machine health monitoring in applications where runtime is very important or downtime is very expensive, and is done by instrumenting machines (e.g., pressurized air lines, water flow) and feeding the data to a machine learning algorithm. More recently, generative AI has been auditioned for chatbots on city websites.
With enough data, good ML will surely find anomalies, and advance over time to become more useful. Today, though, there are limitations and challenges and it is paramount that we keep these in mind as we attempt to apply AI and machine learning.
Limitations and Challenges with AI/ML in the Marketplace
- It Ain’t Broke[TM]. Human engineers are really pretty good at avoiding and detecting impending problems. Routine maintenance is performed so that, at the very least, someone periodically has hands and ears on a motor, for instance. And the ear is a reasonably good diagnostic tool in the case of running motors. It’s not the only one (just a very simple one a lot of people have!).
- It Ain’t Broke, Part 2[TM]. ML needs big data sets, preferably with lots of clear failure examples, in order to analyze and be able to identify the failures in data before they occur. Yet, the kinds of motorized machines we are discussing tend to run well for a long time. Anomalies are rare in reliable industrial systems, and what tends to look like an anomaly is probably benign: spin-up, shutdown, and normal electrical variances. Gathering enough data to apply ML could take years.
- The Economics of Software Development Doesn’t Lend to the Needs of AI/ML. Software product managers prioritize developer time to complete the maximum amount of products. They look for achievable, low-risk stuff. AI/ML algorithms tend to be complex, relatively high-risk to code, and even more complex to test.
- Software must pass QA/QC tests. Does the product perform as advertised? Always? AI algorithms are difficult for Quality Assurance (QA) and Quality Control (QC) by design. How they derive answers can be inscrutable and even non-repeatable.
- The End User Wants Monotony. Facility and city managers want everything ideally to be reliable, predictable, and boring. They need to be able to understand or at least trust any software’s behavior. That’s tough to do with AL/ML by its very nature.
What is the Future of AI/ML in Smart Cities?
So the state of AI/ML today has some challenges, but the pace of AI/ML development is an all-out sprint. It’s difficult to know when or where we will see solid use cases, but SRT Labs sees roles for it in the data richness and analytics demands of:
- machine health monitoring,
- energy flows into and within facilities (monitoring and control),
- Traffic flow monitoring and control,
- Concierge chatbots and actual physical robots,
- Security, which will be integrated into physical robots,
- Occupancy sensing optimized for privacy over efficiency.
Please engage in the discussions on the growth of AI/ML! Engage those who are already working in the field and those who will be applying the outcomes. Tracking the developments and limitations of the tools (aside from the marketing) will be critical so that we use and apply tools that are safe for everyone.